Are Traditional Stock Merging Methods Dead? Find Out How Machine Learning is Taking Over!
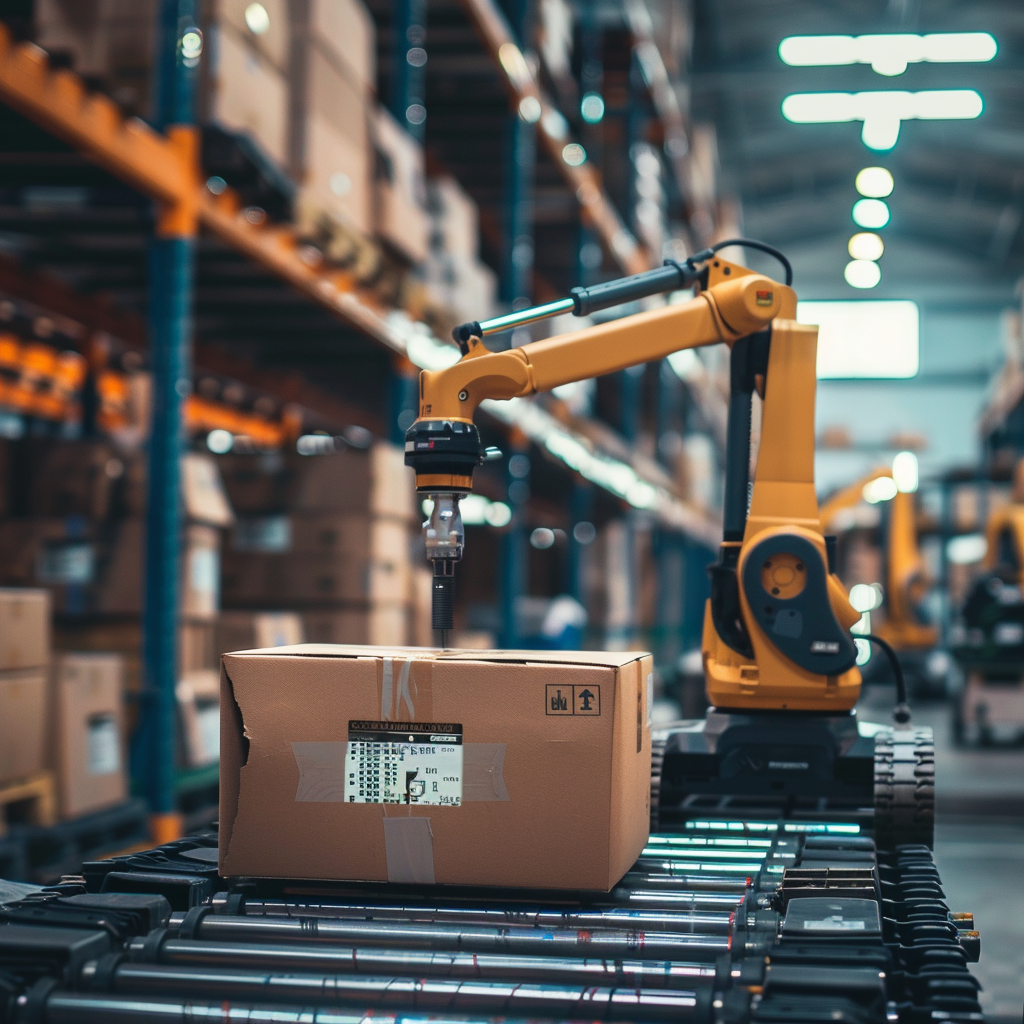
Predictive analytics and machine learning are revolutionizing stock merging processes, with firms utilizing these technologies seeing a 10-20% increase in returns compared to traditional methods (Deloitte, 2023). Let's learn how the transformative impact of these technologies on stock merging.
Pattern and Trends Recognition: Machine learning algorithms have been at the forefront of this technological shift. Analyzing vast amounts of historical and real-time data, these algorithms can identify patterns and trends that are often invisible to human analysts. This significant improvement underscores the potential of machine learning to optimize stock merging processes.
Accelerated Decision-Making: According to a report by McKinsey & Company, financial firms that adopt advanced data analytics can reduce their decision-making time by up to 30% (McKinsey, 2022). This reduction in time is crucial in the fast-paced world of stock trading, where timely decisions can mean the difference between significant gains and substantial losses.
Improved Risk Management: Machine learning models can forecast potential market downturns by analyzing indicators such as market sentiment, economic indicators, and historical price movements. For example, during the COVID-19 pandemic, machine learning models that incorporated pandemic-related data outperformed traditional models by 15%, providing more accurate predictions of market behavior (Financial Times, 2021). This capability allows investors to mitigate risks and make more informed decisions.
Development of Algorithmic Trading: This approach uses pre-programmed instructions based on multiple market variables to execute trades at optimal times. According to a report by Statista, algorithmic trading accounted for approximately 60-73% of all equity trading in the United States in 2020 (Statista, 2021). This high adoption rate reflects the effectiveness of machine learning algorithms in executing trades with minimal human intervention, thereby reducing errors and increasing efficiency.
Integration of Diverse Data Sources: A study by the Massachusetts Institute of Technology (MIT) demonstrated that incorporating social media sentiment analysis into trading algorithms improved the accuracy of stock price predictions by 8% (MIT, 2022). This integration enables a more comprehensive analysis of market dynamics, providing traders with a holistic view of factors influencing stock prices.
In conclusion, the integration of predictive analytics and machine learning in stock merging processes offers a transformative approach to stock trading. By leveraging advanced algorithms and vast datasets, financial institutions can enhance their decision-making processes, manage risks more effectively, and achieve better trading outcomes. The statistics and real-world examples cited in this paper underscore the significant impact of these technologies on the financial sector, highlighting their potential to revolutionize stock merging and trading strategies.
Sources
- Deloitte. (2023). Machine learning in trading: How it improves financial returns.
- Financial Times. (2021). How machine learning models performed during the COVID-19 pandemic. Financial Times.
- Massachusetts Institute of Technology. (2022). Impact of social media sentiment on stock price predictions. MIT News.
- McKinsey & Company. (2022). Advanced analytics in financial services. McKinsey & Company. Retrieved from https://www.mckinsey.com/business-functions/mckinsey-analytics/our-insights/how-advanced-analytics-can-improve-decision-making-in-financial-services
- Statista. (2021). Algorithmic trading in the United States. Statista.