Is Adopting ML Worth It for Your Business?
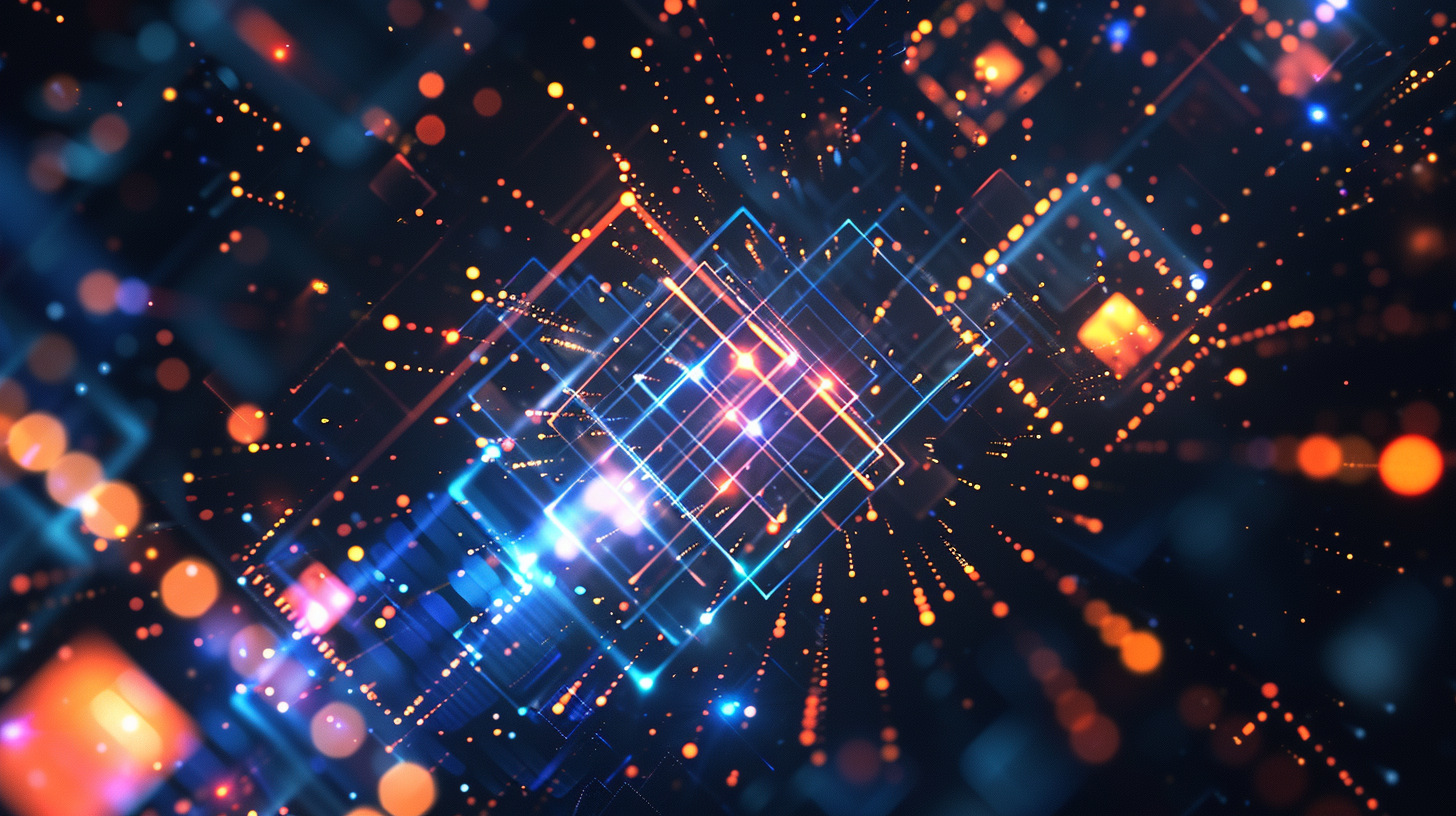
Artificial intelligence (AI) is the vast field of creating intelligent machines. Machine learning (ML) is a powerful technique within AI that allows machines to learn from data and improve on their own. Understanding their differences is key to unlocking ML's true potential.
Discussion about AI and ML
At its core, AI revolves around the notion of intelligent agents navigating and influencing their surroundings to accomplish predefined objectives. Unlike traditional software programs that follow rigid instructions, AI agents possess the ability to adapt, learn from experience, and improve their performance over time. This dynamic interplay between humans and machines prompts profound questions about consciousness, ethics, and the future of human-machine collaboration.
ML, as a subset of AI, plays a pivotal role in realizing the potential of artificial intelligence across various domains. By leveraging algorithms and statistical models, ML enables systems to learn from data, identify patterns, and make informed decisions without explicit programming. This capability unlocks a myriad of possibilities, from engineering intelligent entities to revolutionizing sectors like healthcare, business analytics, and beyond.
Technological Advancements
Businesses can leverage ML frameworks like TensorFlow and PyTorch, along with AI services from cloud providers such as AWS, Google Cloud, and Microsoft Azure. These tools facilitate the integration of ML and AI into existing systems, enhancing their capabilities.
Yet, it's essential to maintain a holistic perspective on AI and ML, recognizing their limitations and the evolving landscape of computational technology. While digital computing has been the primary substrate for ML thus far, emerging paradigms such as quantum, analog, optical, and biological computing hold promise for expanding the horizons of artificial intelligence.
Impact on Business Processes
AI-driven predictive analytics can forecast market trends and consumer behavior, enabling businesses to stay ahead of the competition. Additionally, chatbots powered by natural language processing (NLP) can handle customer inquiries 24/7, improving customer satisfaction and reducing operational costs.
In essence, ML serves as a catalyst for AI advancement, laying the groundwork for a future where humans and machines collaborate seamlessly to tackle complex challenges and achieve shared goals. By harnessing the power of data and computational algorithms, ML empowers AI agents to learn, adapt, and evolve in a rapidly changing world. As we navigate this journey towards human-compatible AI, it's imperative to approach AI development with wisdom, foresight, and a deep appreciation for the symbiotic relationship between humans and machines.
Practical Applications of Machine Learning
As theoretical as some machine learning model use cases can seem—like driverless cars—plenty of them are commonplace. Here are some of the most well-known examples:
1. Web Search
Tools like Google and Bing can deliver more than a billion results for a search term in less than a second thanks to ML. ML ensures that search engines can process misspelled or grammatically incorrect queries and still deliver relevant results. Additionally, through natural language processing (NLP), voice-activated search can be just as accurate.
2. Customer Service
Early chatbots could only answer basic questions with a selection of preset responses before requiring a human customer service agent to intervene. Today's advanced chatbots, powered by ML models, can resolve a wide range of issues. They utilize historical conversation data—not just from an individual's record but also from similar queries by other customers—to ensure appropriate responses, thereby boosting customer satisfaction and maintaining brand loyalty.
3. Recommendation Engines
E-commerce platforms like Amazon and streaming services like Netflix use ML-powered recommendation engines. These engines analyze user data to form profiles based on preferences and generate personalized recommendations. The more users interact, the more accurate these recommendations become, enhancing user experience and driving engagement.
4. Fraud Detection
Credit card companies utilize ML algorithms to analyze transaction patterns and detect anomalies that may indicate fraud. When unusual transaction patterns are identified, the ML system can recommend or initiate a lock on the card, prompting the holder to verify the activity, thus preventing potential fraud.
5. Medical Diagnostics
Cutting-edge medical tools leverage ML to derive insights from raw image data. For instance, MIT researchers developed an ML model in 2021 capable of predicting cancer risk with high accuracy. Such advancements hold promise for early detection and treatment of life-threatening conditions, especially significant given the aging population and prevalence of late-life diseases.
Case Study: Amazon's Omnichannel and ML Mastery
Many retailers are adopting omnichannel strategies to meet customer expectations and gain a competitive edge. A great example is Amazon, a trillion-dollar company that has masterfully leveraged customer-centric innovation to propel its growth. By building its organization around artificial intelligence (AI) and machine learning (ML), Amazon has become the largest e-commerce player in the world, and it continues to innovate and dominate the market.
Here’s how Amazon successfully integrates ML into its omnichannel strategy:
Personalized Product Recommendations:
Amazon practically invented personalized recommendations by using sophisticated ML that analyzes a vast amount of data to offer the right product at the right time. This approach drives 35% of Amazon's total purchases.
The recommendation engine collects data on buying behavior, cart items, viewed products, and frequently searched items, and uses item-to-item collaborative filtering to generate personalized suggestions.
ML-Optimized Search Relevancy:
Amazon's ML-driven search bar significantly increases conversion rates. For instance, customers who use the search bar have a 42% chance of making a purchase, compared to 16% for Walmart and 13% for Etsy.
The A9 algorithm (now A10) emphasizes sales conversions, leading to a nearly 6x increase in conversion rates when customers use the search function.
Alexa-Enabled Voice Shopping:
Alexa, Amazon's ML assistant, supports voice shopping, allowing users to search for products, add them to the cart, and complete purchases hands-free. Alexa devices account for 26.4% of the global smart speaker market, showcasing Amazon's dominance in this space.
This ML -powered voice shopping provides convenience and personalized reminders, further solidifying Amazon’s market position.
ML in Warehouse and Supply Chain Optimization:
Amazon uses AI to predict consumer demand, manage inventory, optimize delivery routes, and track the supply chain. This integration has enabled innovations like one-day shipping and the use of automated drones for quicker delivery.
AI has been instrumental in expanding and streamlining Amazon’s global operations, enhancing efficiency and customer satisfaction.
Amazon Go Stores with ‘Just Walk Out’ Technology:
Amazon Go stores use ML to eliminate checkout lines, allowing customers to simply walk out with their purchases. This is achieved through computer vision, sensor fusion, and deep learning, which track items taken from or returned to shelves.
This technology, first launched in a Seattle convenience store, has expanded to 32 stores in the US and 15 in the UK, setting a new standard for retail convenience.
Key Challenges and Limitations
Despite its benefits, ML faces notable challenges. Ethical concerns, particularly around data privacy and bias, are significant. For example, biases can emerge if ML models ingest data reflecting systemic inequalities. Additionally, the effectiveness of ML models is highly dependent on data quality; errors in metadata can lead to significant inaccuracies.
- Ethical Concerns: Data privacy advocates and lawmakers have raised concerns over ML and AI ethics, leading to bans on technologies like facial recognition in various jurisdictions. Additionally, biases in ML models, stemming from inequitable data, can result in discriminatory outcomes.
- Data Quality: ML models are only as effective as the data they are trained on. Ensuring high data quality and pre-tagging data sets for supervised learning are critical steps in minimizing errors.
- Programming Challenges: ML models require careful programming. Even minor glitches can cause significant errors, emphasizing the need for meticulous oversight during the development phase.
Future Trends and Practices
1. The Role of Cloud Analytics
The future of ML looks promising, especially with advancements in cloud technologies. Cloud analytics allows organizations to manage data processing and storage off-premises, facilitating faster and more efficient data analysis. As cloud technologies advance, using ML for analytics within cloud deployments will unlock more valuable insights at a quicker pace.
2. AI and ML in Healthcare
AI and ML will continue to revolutionize healthcare, improving medical diagnosis, drug discovery, and personalized treatment plans. According to Allied Market Research, the global healthcare AI market is projected to reach $19.25 billion by 2026, with a CAGR of 43.5% from 2019 to 2026.
3. AI and ML in Retail
Retailers will increasingly leverage AI and ML to enhance customer experience, boost sales, and optimize inventory management. Businesses using AI and ML have reported a 40% increase in customer loyalty and a 35% increase in customer satisfaction, according to Salesforce.
4. AI and ML in Finance
Financial institutions will use AI and ML to improve fraud detection, risk management, and investment strategies. The global AI in banking market is projected to grow to $64.03 billion by 2030, at a CAGR of 32.6% during 2021-2030, as per ResearchAndMarkets.com.
5. AI and ML in Manufacturing
Manufacturing will benefit from AI and ML through improved supply chain management, reduced downtime, and optimized production processes. The global AI in manufacturing market is expected to grow from $1.1 billion in 2020 to $16.7 billion by 2026, with a CAGR of 57.2%, according to MarketsandMarkets.
Careful oversight is necessary to gain as much business value from ML as possible while minimizing the effects of its limitations. In addition to being vigilant for bias and data quality, and ensuring models are carefully programmed at the outset of ML initiatives, your enterprise will need to carefully manage the data associated with—and generated by—these projects.
Conclusion
In conclusion, the synergy between AI and ML epitomizes the transformative potential of technology in shaping the future of humanity. By understanding the intricate interplay between these disciplines, we can harness their collective power to drive innovation, solve pressing problems, and create a more equitable and sustainable world for generations to come.
Egitech – Your Tech Buddy
At Egitech, we address critical business pain points such as data overload and inefficient processes by integrating AI and ML technologies. Our Cloud & AI Consulting, along with IaaS, PaaS, SaaS, and MLaaS offerings, provide scalable solutions tailored to your needs. By leveraging advanced data processing, automation, and predictive analytics, we transform inefficiencies into growth opportunities, enabling informed decision-making and enhanced operational efficiency.