Machine Learning: The Unseen Force Protecting Your Money!
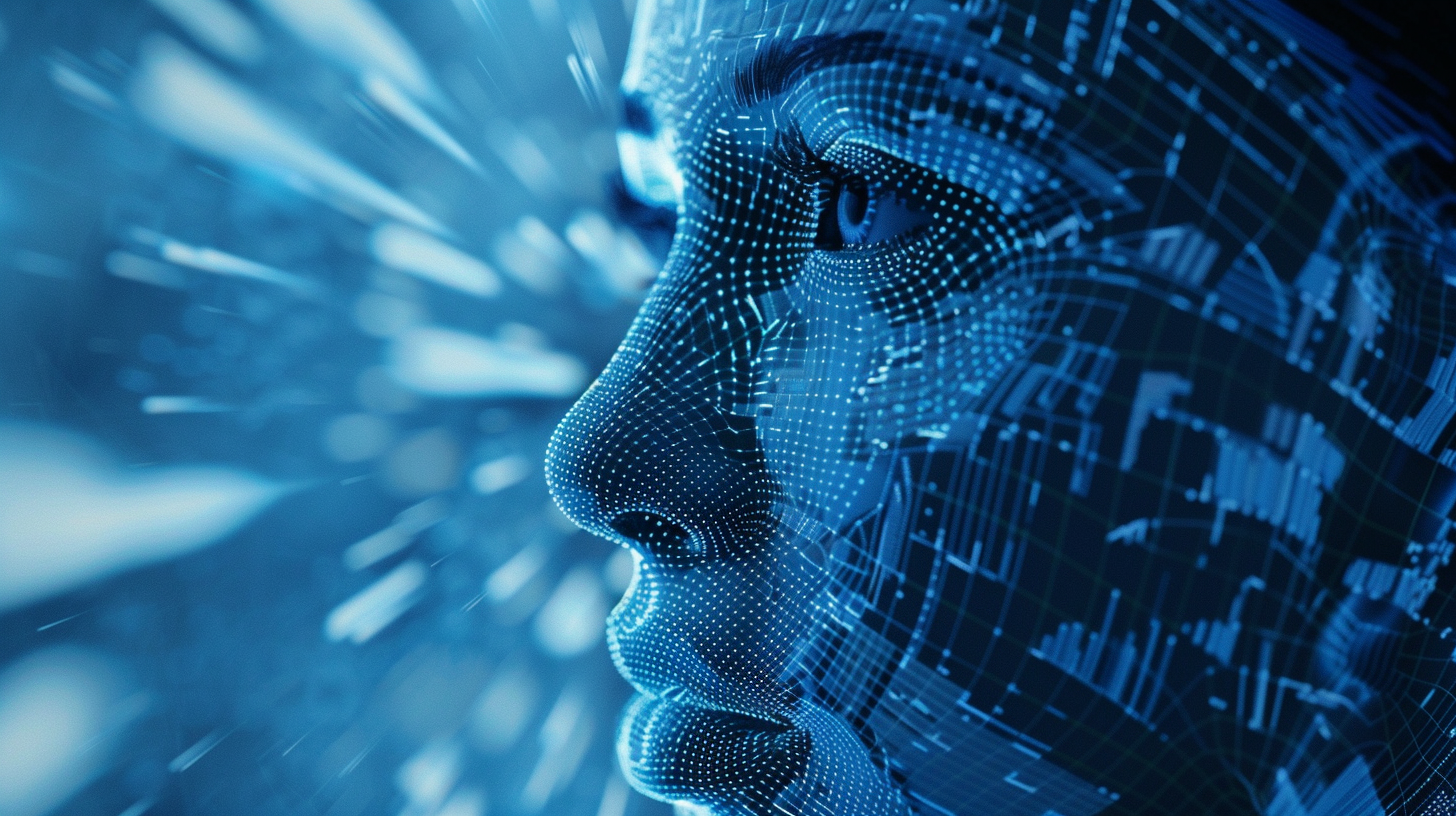
Machine learning turns financial data into a fraud-fighting superpower by spotting sneaky patterns and adapting to new tricks, here are some ways it detects fraudsters in the act.
1. Recent statistics underscore the impact of ML in fraud detection:
- According to a 2023 survey by Juniper Research, businesses using ML-based fraud detection systems reported a 41% decrease in fraudulent activities compared to those relying on traditional methods.
- A 2024 Gartner study highlighted that over 75% of companies with mature ML models for fraud detection experienced a reduction in fraud-related losses by at least 30%.
- Researchers found that integrating ML and big data analytics reduced fraudulent transactions by 35% within six months of implementation in major e-commerce platforms (Reddy et al., 2024).
- Global Fraud Losses: According to a report by the Association of Certified Fraud Examiners (ACFE), global fraud losses reached $4.7 trillion in 2022, highlighting the pervasive nature of fraud across various industries (ACFE, 2023).
2. The ML Application for Fraud Detection
Data Collection and Preprocessing
Data collection is the foundational step in developing an ML-based fraud detection system. Retailers gather data from various sources, including transaction records, customer profiles, and historical fraud cases. Ensuring the quality and variety of this data is crucial for the effectiveness of the ML models.
Preprocessing involves cleaning and formatting the data to make it suitable for analysis. This includes handling missing values, normalizing data, and removing duplicates. Techniques such as data augmentation can also be employed to create a more robust dataset.
Feature Extraction
Feature extraction is the process of identifying key attributes from the dataset that are indicative of fraudulent behavior. These features could include transaction amounts, frequency of returns, and geographic locations of purchases.
Advanced techniques such as Principal Component Analysis (PCA) and Feature Selection algorithms are used to extract and select the most relevant features. This step is critical as it directly impacts the performance of the ML model by focusing on the most informative aspects of the data.
Rule Definition and Management
Rule definition involves creating initial guidelines based on known fraud indicators. These rules serve as a baseline for the ML models and are defined by domain experts who understand the intricacies of fraud patterns.
Management of these rules is an ongoing process. As new fraud patterns emerge, the rules are updated and refined. This iterative process ensures that the ML models remain effective in detecting evolving fraud tactics.
Model Training and Evaluation
Training ML models involves using historical data to teach the algorithms how to identify fraud. Various algorithms, such as decision trees, random forests, and neural networks, are employed to train the models.
Evaluation of the models is done using metrics like accuracy, precision, recall, and F1-score. These metrics help in assessing the model's performance and its ability to correctly identify fraudulent transactions while minimizing false positives.
Integration and Deployment
Once trained and evaluated, the ML models are integrated into the retailer's systems. This integration allows the models to operate in real-time, continuously monitoring transactions and flagging suspicious activities.
Deployment also involves continuous monitoring and updating of the models. As new data is collected, the models are retrained to adapt to new fraud patterns. This ensures that the fraud detection system remains effective and up-to-date.
In short, Machine Learning significantly enhances fraud detection by processing large volumes of data in real-time, identifying complex patterns, and continuously improving its detection mechanisms. The future of ML in fraud prevention looks promising with advancements in deep learning, federated learning for enhanced privacy, and improved model interpretability. Through leveraging ML, retailers can achieve substantial cost savings, reduce fraud losses, and increase operational efficiency, making it an indispensable tool in modern fraud detection.
Beyond finance, what other industries could leverage machine learning to combat fraud and create a safer digital landscape?
With the increasing importance of data privacy, how can we ensure that ML-powered fraud detection remains ethical and protects consumer information?
Sources
- Association of Certified Fraud Examiners (ACFE). (2023). Report to the Nations: 2022 Global Study on Occupational Fraud and Abuse.
- Gartner. (2024). Fraud Detection and Prevention Market Study.
- Juniper Research. (2023). Impact of ML-based Fraud Detection on Businesses.
- MarketsandMarkets. (2022). Fraud Detection and Prevention Market - Global Forecast to 2026.