Top 7 Machine Learning Use Cases Revolutionizing Logistics
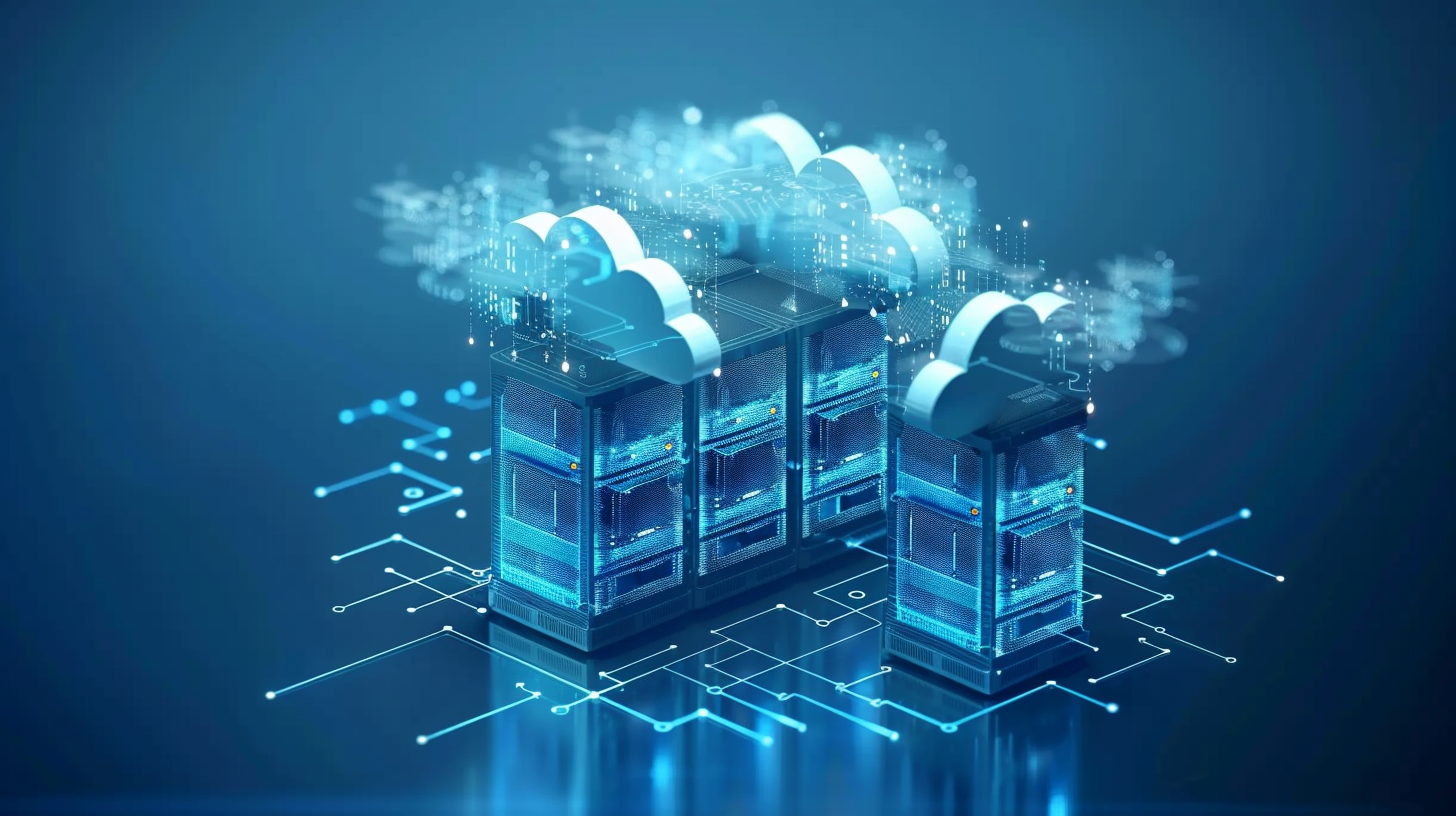
According to McKinsey, implementing AI has helped companies improve logistics costs by 15%, inventory levels by 35%, and service levels by 65%. Besides, enhanced delivery accuracy and speed through machine learning can improve customer satisfaction ratings by 10-15%. Let’s explore various use cases of ML in Logistics.
Inventory Management
Managing inventory manually is costly and challenging, often affecting cash flow and profit margins. ML revolutionizes inventory management by accurately predicting demand, reducing carrying costs, and minimizing stockouts. Effective implementation of ML in inventory management can improve demand forecasting accuracy by 30-40%, leading to better inventory management. However, effective forecasts require large datasets. To address this, ML employs methods such as data augmentation, incremental learning, and reinforcement learning to improve data diversity and accuracy.
- Data Augmentation: Techniques like SMOTE for numerical data or neural network-generated data for unstructured data enhance the dataset without new data collection.
- Incremental Learning: Starts with a simple model and improves as more data becomes available, continuously refining predictions.
- Reinforcement Learning: Uses reward and punishment to optimize the system’s behavior over time.
Choosing the right data storage solution, like data lakes for in-depth analysis or data warehouses for operational processes, is critical for effective ML implementation.
Warehouse Management
ML plays a crucial role in automating tasks, anticipating challenges, and reducing paperwork in warehouses. Technologies like computer vision and NLP enable real-time monitoring and automatic recognition of packages, enhancing efficiency. Implementing machine learning in warehouse operations can increase productivity by 20-30%. ML-powered autonomous vehicles and robots streamline operations such as receiving, packing, and transporting goods, contributing to safer and more productive warehouses.
Predictive Maintenance
Predictive maintenance uses ML algorithms to analyze data and predict when maintenance is needed, preventing issues before they occur. This proactive approach reduces downtime, extends equipment lifespan, and identifies potential problems early. Implementing predictive maintenance with machine learning can reduce maintenance costs by 10-15%.
Route Optimization
Route optimization is vital in logistics for determining the most efficient paths for deliveries. AI analyzes data from traffic sensors, weather forecasts, and GPS to build optimal routes, reducing fuel costs and delivery times. Machine learning algorithms can reduce delivery times by optimizing routes and schedules, potentially cutting them by 20-25%. This leads to cost savings and a better customer experience.
Back Office Operations
Back-office tasks such as writing emails, processing documents, and managing schedules can be automated using AI, freeing up employees to focus on more critical activities. AI systems handle document automation, data input, scheduling, package tracking, and email generation, increasing overall efficiency. Implementing machine learning in logistics can lead to operational cost savings of up to 10%.
Dynamic Pricing Models
As supply chains become more complex, dynamic pricing strategies driven by AI help logistics companies adapt prices based on factors like supply and demand, competitor pricing, and customer behavior. AI-based dynamic pricing software enables real-time monitoring and adjustment of prices, increasing profitability and agility.
Demand Forecasting
Accurate demand forecasting is crucial for optimizing inventory levels and improving supply chain planning. AI-powered systems provide precise sales predictions, reduce waste and stockouts, and enhance supply chain operations. This capability helps e-commerce businesses meet customer demands more effectively. Implementing machine learning can improve demand forecasting accuracy by 30-40%.
Conclusion
Machine learning significantly enhances various aspects of logistics, from inventory and warehouse management to predictive maintenance and route optimization. By addressing key challenges and leveraging advanced analytics, ML provides logistics companies with the tools to improve efficiency, reduce costs, and enhance customer satisfaction. As technology continues to advance, the role of ML in logistics will only grow, driving innovation and transforming the industry.